ForkGAN: Seeing into the Rainy Night
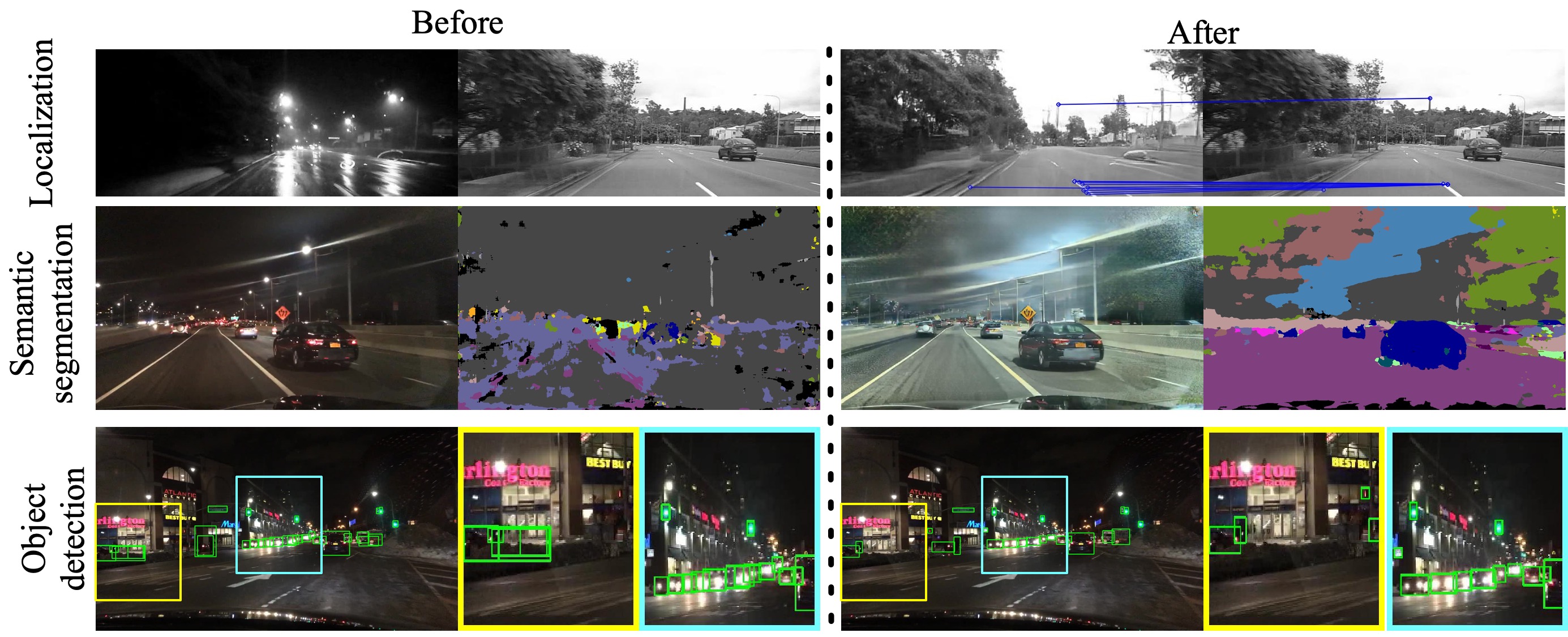
Abstract
We present a ForkGAN for task-agnostic image translation that can boost multiple vision tasks in adverse weather conditions. Three tasks of image localization/retrieval, semantic image segmentation, and object detection are evaluated. The key challenge is achieving high-quality image translation without any explicit supervision, or task awareness. Our innovation is a fork-shape generator with one encoder and two decoders that disentangles the domain-speci c and domain-invariant information. We force the cyclic translation between the weather conditions to go through a common encoding space, and make sure the encoding features reveal no information about the domains. Experimental results show our algorithm produces state-of-the-art image synthesis results and boost three vision tasks' performances in adverse weathers.
Architecture
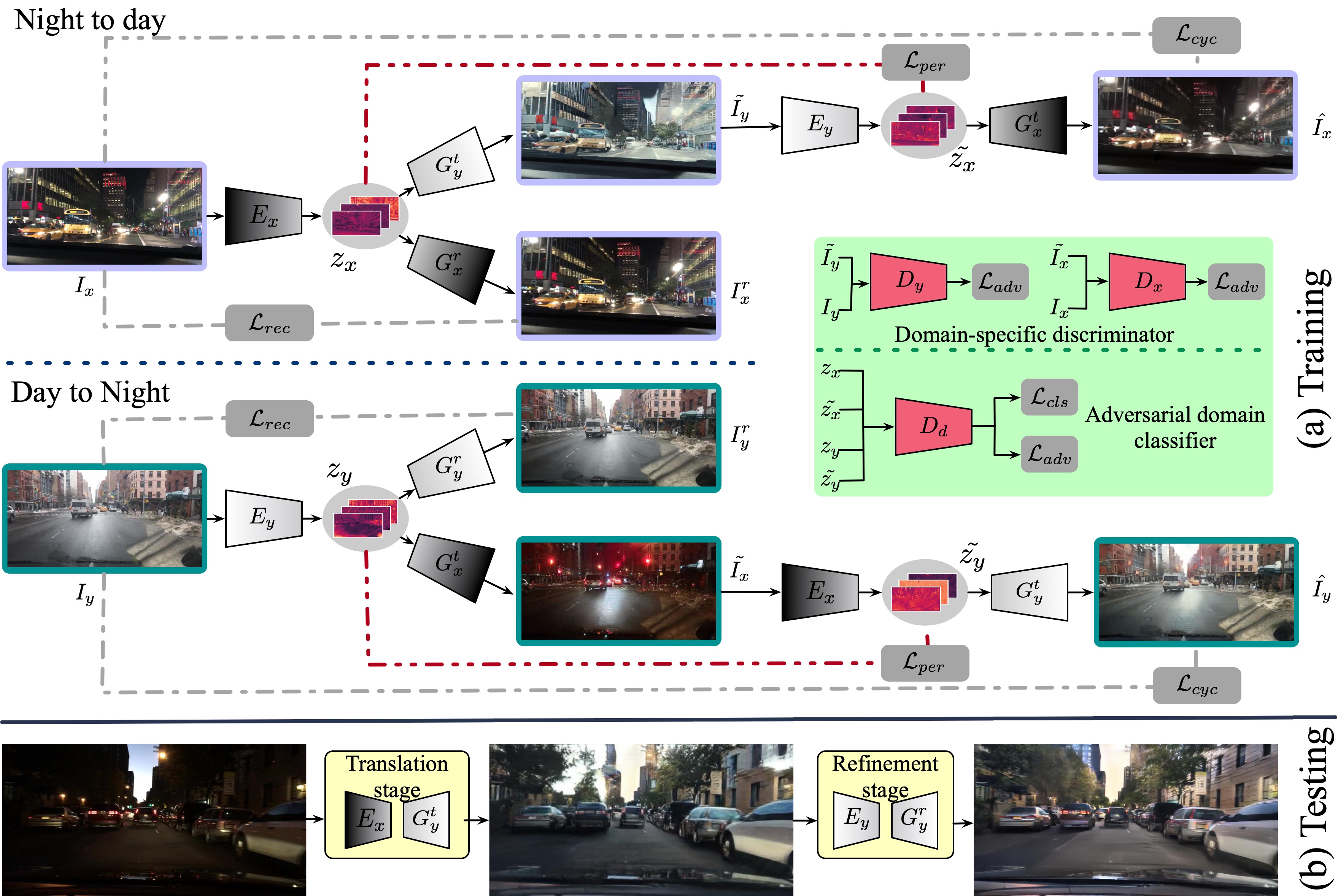
BDD100K result
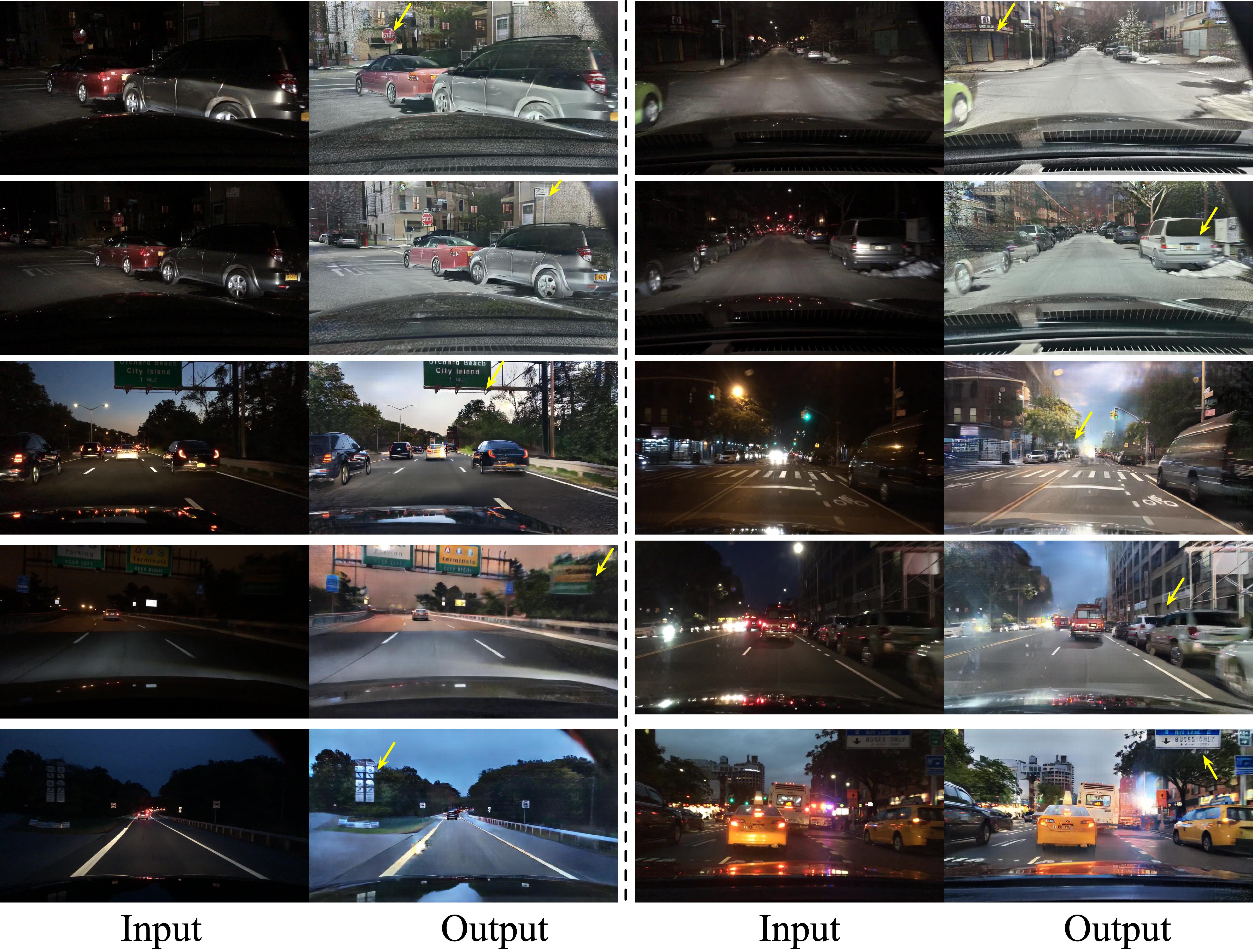
Video presentation
Before the image translation, the semantic segmentation cannot obtain a precise semantic perception output.
Thus, we proposed our ForkGAN to do the image translation. Then we perform semantic segmentation on the enhanced outputs to obtain consistent and accurate outputs
More results (BDD100K dataset)
Citation
@inproceedings{zheng2020forkgan, title={Forkgan: Seeing into the rainy night}, author={Zheng, Ziqiang and Wu, Yang and Han, Xinran and Shi, Jianbo}, booktitle={European Conference of Computer Vision}, pages={155--170}, year={2020}, organization={Springer} }